:Your Personalized Image Animator
via Plug-and-Play Modules in Text-to-Image Models
Merry Christmas!
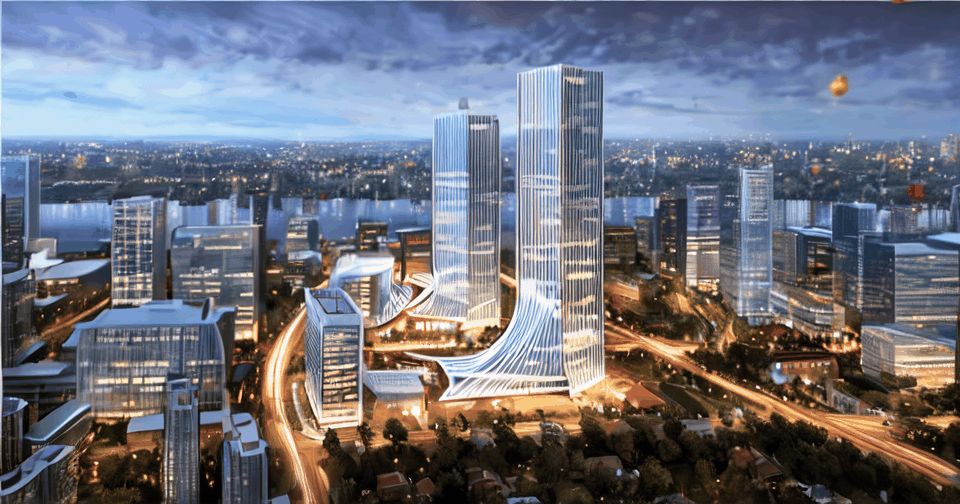
PIA is a Personalized Image Animator that excels in aligning with condition images, achieving motion controllability by text, and the compatibility with various personalized T2I models without specific tuning.
Given an elaborated image generated by a personalized text-to-image model, the proposed PIA animates it with realistic motions according to different text prompts while preserving the original distinct styles and high-fidelity details.
Recent advancements in personalized text-to-image (T2I) models have revolutionized content creation, empowering non-experts to generate stunning images with unique styles. While promising, adding realistic motions into these personalized images by text poses significant challenges in preserving distinct styles, high-fidelity details, and achieving motion controllability by text. In this paper, we present PIA, a Personalized Image Animator that excels in aligning with condition images, achieving motion controllability by text, and the compatibility with various personalized T2I models without specific tuning. To achieve these goals, PIA builds upon a base T2I model with well-trained temporal alignment layers, allowing for the seamless transformation of any personalized T2I model into an image animation model. A key component of PIA is the introduction of the condition module, which utilizes the condition frame and inter-frame affinity as input to transfer appearance information guided by the affinity hint for individual frame synthesis in the latent space. This design mitigates the challenges of appearance-related image alignment within and allows for a stronger focus on aligning with motion-related guidance.
PIA consists of a text-to-image (T2I) model, well-trained temporal alignment layers (TA), and a new condition module Wcond responsible for encoding the condition image zI and inter-frame affinity s{1:F}. In particular, the T2I model consists of U-Net blocks, including a ResBlock (Res), a self-attention layer (SA), and a cross-attention layer (CA), as depicted in (b). During training, the condition module learns to leverage the affinity hints and incorporate appearance information from the condition images, facilitating image alignment and enabling a stronger emphasis on motion-related alignment.
Make your animation with our open source code or online demo.
@article{zhang2023pia,
title={PIA: Your Personalized Image Animator via Plug-and-Play Modules in Text-to-Image Models},
author={Yiming Zhang and Zhening Xing and Yanhong Zeng and Youqing Fang and Kai Chen},
year={2023},
eprint={2312.13964},
archivePrefix={arXiv},
primaryClass={cs.CV}
}